January 8, 2020
Incremental Lift Calculation: How Many Incremental Conversions Did You Gain?
Nick Schenck
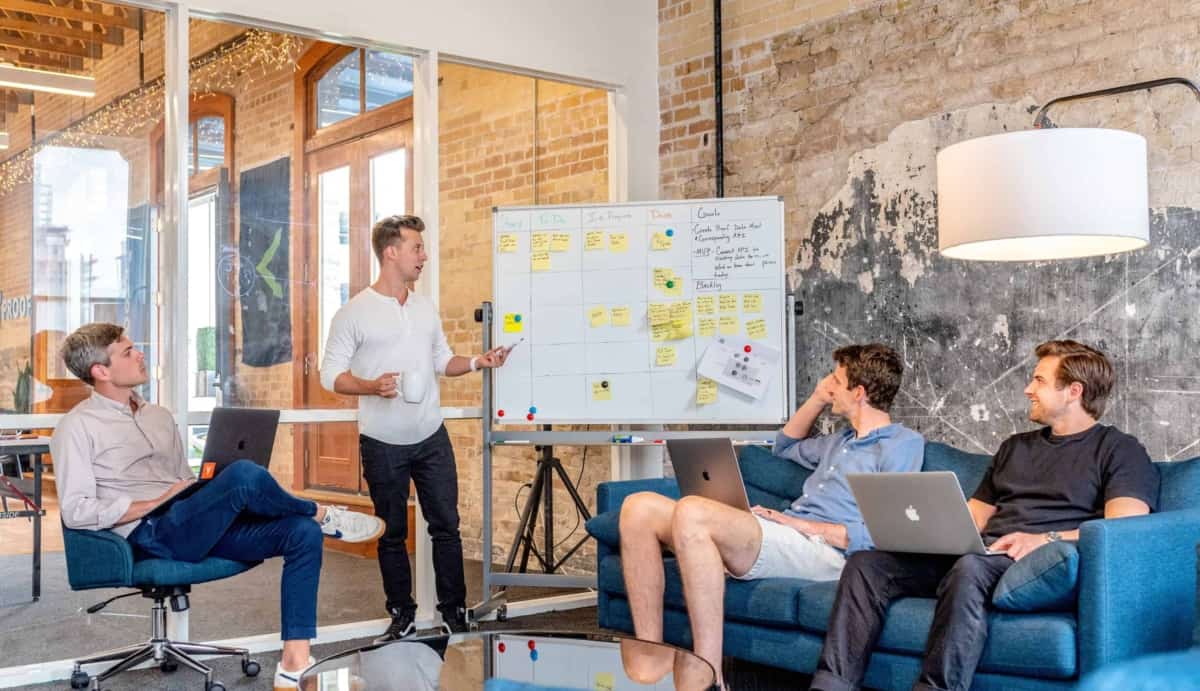
The toughest question for marketers to answer is how many incremental sales their ad campaigns generate.
When Google, Facebook, YouTube, Bing, etc. takes credit for driving sales for your company, are you confident that these sales wouldn’t have occurred otherwise?
We’ve developed a methodology to help answer this question. It’s not perfect, but it may work for your business.
First, here are some nuances of the model:
- It accounts mainly for click-thru conversions.
- It works only if you use UTMs, vanity URLs, or purchase codes in your ad campaigns.
- It does not account for all cross-device behavior.
- It is backward-looking, although with enough data, it helps with forecasting.
- It works best if you store conversion data with associated UTM parameters / codes in a database that you can export, but you can also use Google Analytics.
The model is based on these beliefs:
- Ad channels take credit for more conversions than they’re solely responsible for.
- Conversions from return users should be treated differently than those from new users.
- There is no single, best, one-size-fits-all multitouch attribution model.
- View-thru conversions on most ad channels are not worth counting. This is especially true for remarketing campaigns and banner/display ads.
Build A Single Source Of Truth As A Reference
The initial database build is time-consuming, but updating the data each month is fairly straightforward.
In a spreadsheet, build a table that includes:
- Historic month-by-month top-line company sales, revenue, and ad spend dating back as far as is accurate.
- Month-by-month sales and revenue by paid channel separated out by these attribution models:
- Last-click
- First-click
- Position-based
- Linear
- Time-decay
- Last non-direct click
- Channel default
- Average of all attribution models*
- Incremental sales and revenue by ad channel*
The formatting should look something like this below – figures represent dummy data.
*This is explained in subsequent sections.
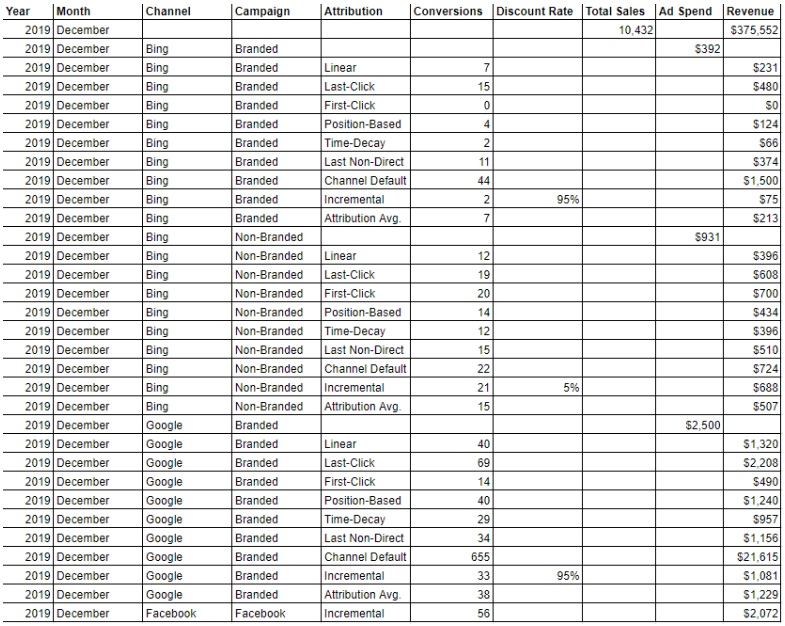
Begin With A Facebook Conversion Lift Test
Most brands can’t run pure holdout tests on any ad channel except for Facebook/Instagram, which has a self-serve model in the Test & Learn section (pictured). Other platforms like YouTube will administer this for you if you have a giant budget.
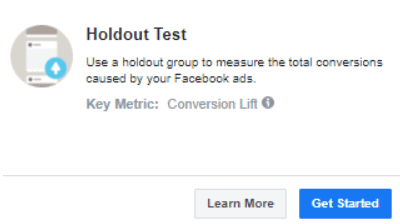
Facebook’s account-level conversion lift test will provide statistically significant data on your incremental cost per acquisition (CPA) and incremental return on ad spend (ROAS) as long as you’re sending that data back to Facebook Ads Manager and you run the test for a long enough duration. These data points are valuable because you can compare these against what is reported at different attribution ranges in Facebook Ads Manager to see how similar the numbers are.
For instance, let’s say the test reveals a $24 incremental CPA. In the same period, Facebook Ads Manager reports a $25 CPA for the 7-day click attribution range. If no other attribution range reports a CPA near $24, this should give you some confidence that the 7-day click range is directionally correct as far as reporting incremental conversion data. That said, I suggest running at least two more account-level holdouts to confirm the results before you put all of your faith behind the 7-day click range.
Assuming the subsequent tests confirm the 7-day click range, you can retroactively see the incremental conversions that your Facebook campaigns yielded in previous months by viewing that 7-day range.* You also can use that 7-day range on an ongoing basis to evaluate the true incremental impact of your campaigns.
You can run the same conversion lift test on specific campaigns (as opposed to an account-level holdout) in your Facebook Ads Manager account. This is helpful if you want to determine (and compare) the incremental CPA and incremental ROAS on remarketing vs. non-remarketing campaigns. The more granular you get, though, the longer it may take to get statistically significant results.
*The assumption is that the test data holds up historically, which may not be the case if your strategy/spend varies significantly, your business is volatile, and if your industry is highly seasonal.
Discount Conversions From Other Ad Channels
Since you can’t determine the incremental conversions on other ad channels as confidently as you can on Facebook, apply a discount rate to the conversions that each channel claims credit for (see below for reference). This discount rate will be based primarily on the channel’s new-to-returning user ratio. The higher the % of return users, the higher the discount rate you should apply.
Why? Because if someone has visited your site, they’re already familiar with your brand, making it less plausible that the advertisement solely influenced their decision to purchase.^ Also, discounting what each channel takes credit for helps to reduce double-counting.
You should also take into account each channel’s default attribution range and lookback window when setting the discount rate. The longer the lookback window, the higher you should set your discount rate because there’s a higher likelihood of other channels (paid, organic, word-of-mouth, etc.) influencing the purchase decision when the window is longer.
Another consideration when you set your discount rates by ad channel is how heavily your campaigns rely on video creative. Videos – more so than display or banner ads – can influence purchases without the user clicking on the ad. Rather than apply a discount rate to view-thru conversions on YouTube non-remarketing, for instance, you can lower the discount rate on click-thru conversions from this channel to account for the presumed influence of your video ads.
This approach also can work for out-of-home campaigns (i.e. radio, billboards, etc.) if you promote a specific vanity URL or discount code in these campaigns. For example, if your radio ad promotes the discount code “BUYNOWRADIO” for 20% off, and your analytics show that 60% of users who purchase using that discount code are return users, it’d be smart to discount those purchases.
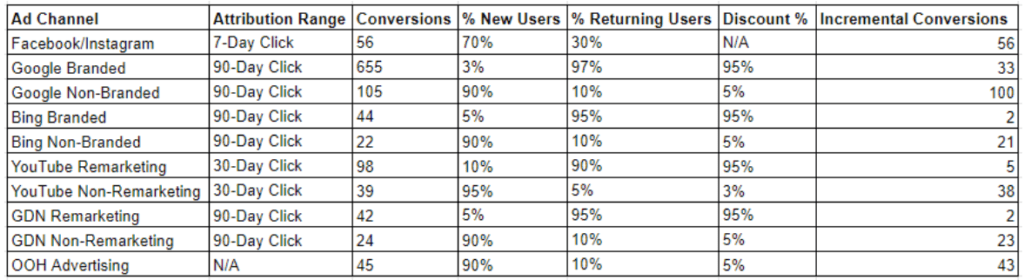
Assigning the discount rates is not a perfect science, but you can adjust the rates to account for your business’ specific use case. For example, if your site has a low homepage-to-funnel conversion rate (i.e. typical for online publishers with a metered paywall), you may want to lower the discount rate on branded search since these types of ads typically lead users directly into the funnel.
^This model has a bias toward the first-touch attribution model, but I am not suggesting that return visitors are discounted entirely. In Google’s first interaction attribution model, the first touchpoint receives 100% of the credit for the sale.
Aggregate The Data And Compare Models
There is one more set of data to add to your spreadsheet before you can pivot and chart the information.
In each month, sum the paid conversions and revenue from each of the attribution models and calculate the month-by-month average.
When you graph all of the data month-by-month, compare each attribution model to the average of all the models and to the incremental paid conversion data.
If your discount rates are in the fairway, so to speak, you should see a high correlation between the monthly avg. model data and the incrementality data. If you notice a high variance, it may indicate that one or more of your discount rates are off.
So why bother with calculating incrementality for each channel when it should map to the avg. of the attribution models? There are several reasons:
- Attribution is imperfect: Attribution assigns credit to channels along the purchase path. It doesn’t compare audience behavior in an A/B test. For instance, pretend you shut down Reddit ad spend. Will this cause sales to decline? Or will sales stay consistent? With attribution models, you won’t know. It’s possible nothing will change except you will see a bump in conversions assigned to a different channel than Reddit. The incremental conversion data is based partly on fact due to the Facebook holdout test, and it is unrelated to where clicks occur along the purchase path. So this data gives you a different way to isolate and evaluate performance by ad channel.
- More data points: The more data you have, the better. Comparing the incremental data with the different attribution models and the average of all attribution models allows you to sanity check your assumptions and triangulate performance.
- Campaign management: Once you feel confident about your incrementality model data, you can apply the discount rates to your ad channel performance to make sure you’re staying within your designated CPA and ROAS targets. This is less cumbersome than averaging out the various attribution models to evaluate ad performance by channel. If you have an agency managing your ad spend, have them report using the discount rates so that they do not pretend your ad performance is better than it actually is.
How To Use The Data To Make Better Decisions
Now that you have a model to reference, what are the advantages to using this?
- Eliminates the veneer: If you take what each ad channel reports at face value, the sum of conversions from paid media may exceed your company’s total top-line sales due to multiple channels claiming credit for the same conversion. Looking at incremental conversions enables you to get real about your aggregate ad channel performance.
- Evens the playing field: Without incremental data, you may incorrectly assume that branded search and remarketing are your best acquisition channels because they have the lowest CPAs and highest ROAS. But when you apply the discount rates to these channels, you can better evaluate these channels compared to so-called “upper funnel” or “brand awareness” channels, which may generate incremental conversions more efficiently.
- Forecasting: Once you are confident about your historical incremental conversions or revenue from paid channels, you can understand what % of total sales (or revenue) your paid channels account for. That knowledge can help you back into your estimated ad spend for future quarters assuming you know the total sales forecast.
- Establishes clear guardrails: You don’t need to ask for a specific ad budget if you reach a consensus internally on what your incremental CPA and incremental ROAS should be. Ideally, you can continue to spend infinitely provided that you remain within those guardrails.
This model is a starting point. There could be valid reasons to spend above an incremental CPA or ROAS target – for instance, if you want to bid higher on branded keywords to deter competitors from bidding on them.
Overall, we find this model to be a useful and sober way to measure and evaluate the impact of ad spend.
If you build this out for your company and have questions, hit us up and we’d be happy to assist.